Minimizing NWP model variation to maximize power forecast accuracy
The accuracy of power forecasts is a vital component in the success of renewable energy power plants. The predicted amount of energy produced needs to be within the smallest error margins possible to ensure the minimum financial penalties and maximum profits to the power plant operator. There are several factors that affect the accuracy of power forecasts, one of them being the quality of numerical weather prediction (NWP) data. Each NWP model has certain strengths and weaknesses depending on conditions such as region, terrain type, and forecast horizon. Knowing these strengths and weaknesses allows making well-informed decisions that can assist in producing forecasts with higher accuracy. Contrarily, overlooking them can lead to poor results.
Bird’s eye view of NWP models thanks to Big Data
In order to analyze these NWP model characteristics, Alexandros Saivanidis has developed a software solution that evaluates the NWP models used for enercast power forecasts at large scale as part of his Bachelors’ thesis, titled “Monitoring the quality of Numerical Weather Prediction models”, that he wrote as a student of the University Kassel during an internship at enercast.
The tool can perform analyses of different weather parameters such as temperature, wind speed or solar irradiance, comparing the respective forecasts of the different NWP models to meteorological station measurements or other actuals. The analyses can be broken down by region or country, forecast horizon (such as intraday or day-ahead forecasts) or terrain type (e.g. high terrain). The required time series data are queried from the enercast MeteoStore X repository, holding 1PB of fast access weather data. Efficiently transforming and processing this large amount of data to create the desired error metrics has been the engineering challenge of the project. These error metrics can then be visualized through a web interface in different charts, e.g. as a histogram showing the error distribution.
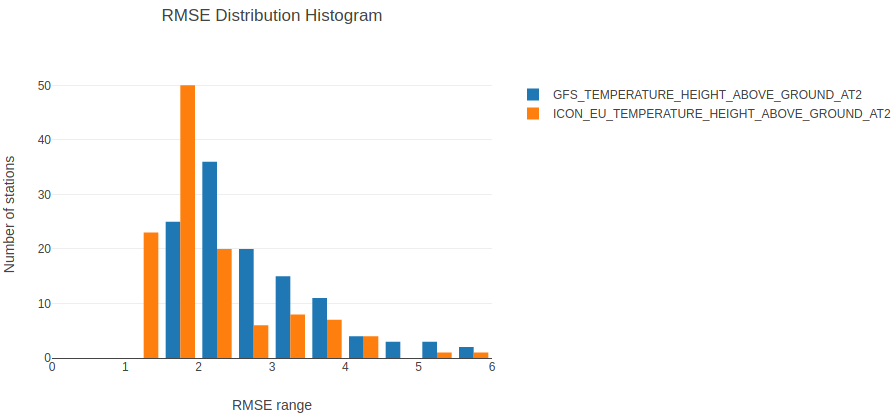
The distribution of the root mean square error (RMSE) of the temperature forecast shows superior quality of the ICON EU-Nest NWP model over the GFS model for 124 high-terrain locations in Germany (forecast horizon: 3 hours ahead, evaluation period: June–August 2020).
Expandability allows to meet customer needs
The tool developed by Alexandros Saivanidis can now be used to inform the product development at enercast. Rather than evaluating power forecasts and weather parameters for single sites, the solution allows for a much faster analysis of the NWP forecast quality at a global scale. This enables enercast to improve forecast accuracy, in particular for new sites where there is not enough data available for site-specific evaluations. In addition, the tool allows enercast to monitor changes in the models’ performance. As the design of the software takes the diversity of customer requirements into account, the evaluation parameters can always be adjusted or extended with new error metrics.
After successfully completing his Bachelor’s thesis, Alexandros Saivanidis has now joined enercast as a full-time application developer. We welcome him to our team!
Get more insights with our Whitepaper
Read our White Paper: Accurate Power Forecasts for Variable Renewable Energy Using enercast e³ Technology to learn more about how consistent evaluations enable your business to get the best possible benefit from your forecasts and which pitfalls you can avoid.